Model-Free Statistical Inference on High-Dimensional Data
报告人:Runze Li,(Pennsylvania State University)
时间:2024-11-18 14:00-15:00
地点:王选报告厅
Abstract: This paper aims to develop an effective model-free inference procedure for high-dimensional data. We first reformulate the hypothesis testing problem via sufficient dimension reduction framework.With the aid of new reformulation, we propose a new test statistic and show that its asymptotic distribution is $\chi^2$ distribution whose degree of freedom does not depend on the unknown population distribution. We further conduct power analysis under local alternative hypotheses. In addition, we study how to control the false discovery rate of the proposed $\chi^2$ tests, which are correlated, to identify important predictors under a model-free framework. To this end, we propose a multiple testing procedure and establish its theoretical guarantees. Monte Carlo simulation studies are conducted to assess the performance of the proposed tests and an empirical analysis of a real-world data set is used to illustrate the proposed methodology.
个人简介:李润泽是美国宾州州立大学统计系讲席教授。他是IMS,ASA和AAAS的fellow。他曾担任统计年刊AOS的联合主编,同时担任JASA等学术期刊副主编。他的荣誉和奖励包括2017年泛华统计学会杰出成就奖,2018年宾州州立大学大学医学院的杰出合作奖,2023年IMS Medalion Lecture, 2023年宾州州立大学大学理学院杰出导师奖及2024年IMS Carver Medal. 他的研究领域包括变量选择和变量筛选,非参数和半参数回归。他的交叉学科研究旨在提升统计学能够更好在社会行为学,神经学和气候研究。
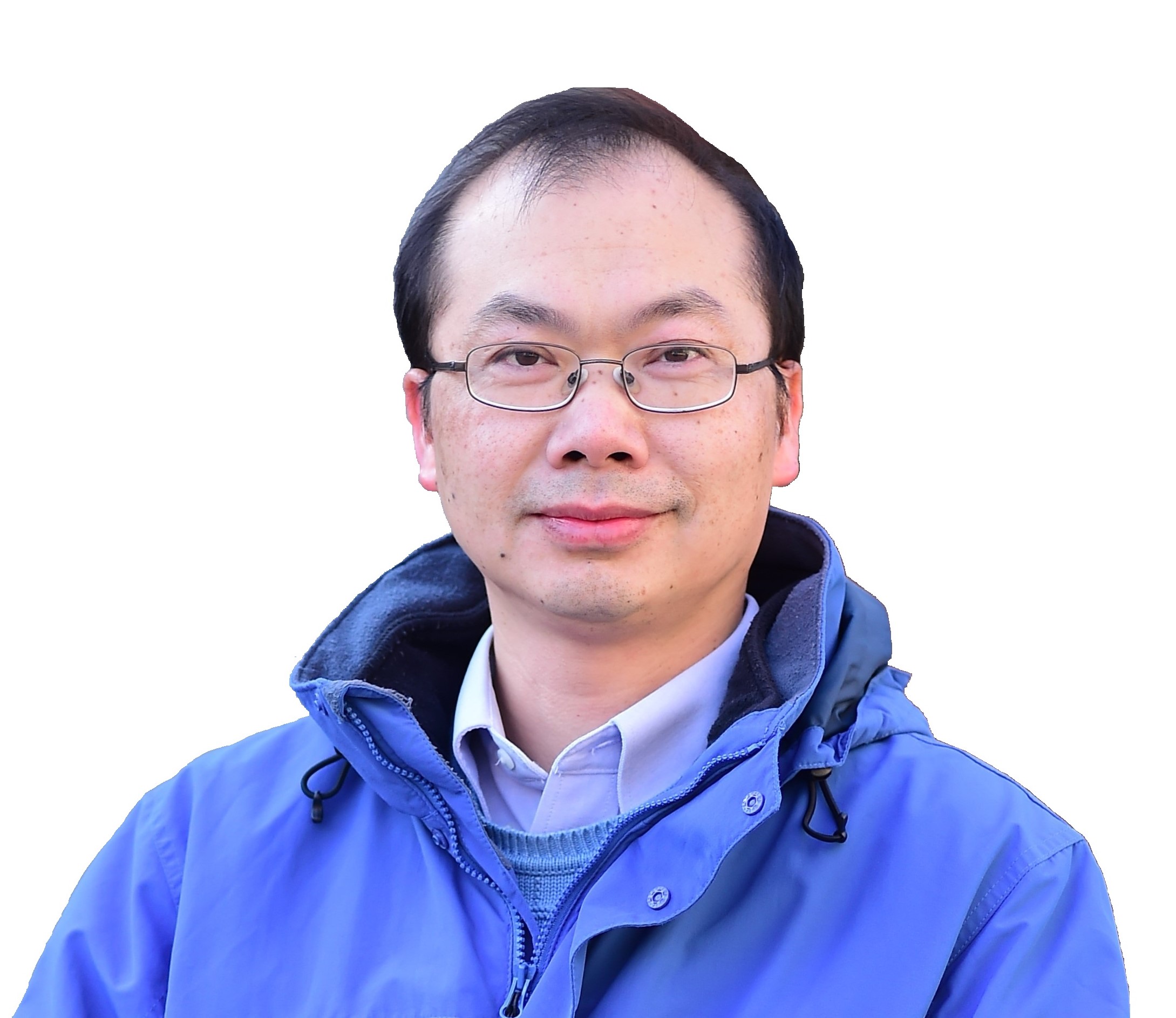